Makeup Like a Superstar: Deep Localized Makeup Transfer Network
Si Liu1 Xinyu Ou1,2,3 Ruihe Qian1,4 Wei Wang1 Xiaochun Cao1
1Chinese Academy of Sciences 2Huazhong University of Science and Technology
3Yunnan Open University 4University of Electronic Science and Technology of China
Introduciton
In this paper, we propose a novel Deep Localized Makeup Transfer Network to automatically recommend the most suitable makeup for a female and synthesis the makeup on her face. Given a before-makeup face, her most suitable makeup is determined automatically. Then, both the before-makeup and the reference faces are fed into the proposed Deep Transfer Network to generate the after-makeup face. Our end-to-end makeup transfer network have several nice properties including: (1) with complete functions: including foundation, lip gloss, and eye shadow transfer; (2) cosmetic specific: different cosmetics are transferred in different manners; (3) localized: different cosmetics are applied on different facial regions; (4) producing naturally looking results without obvious artifacts; (5) controllable makeup lightness: various results from light makeup to heavy makeup can be generated. Qualitative and quantitative experiments show that our network performs much better than the methods of [1] and two variants of NerualStyle [2].
Overview
In our opinion makeup architecture should satisfy five design criteria.
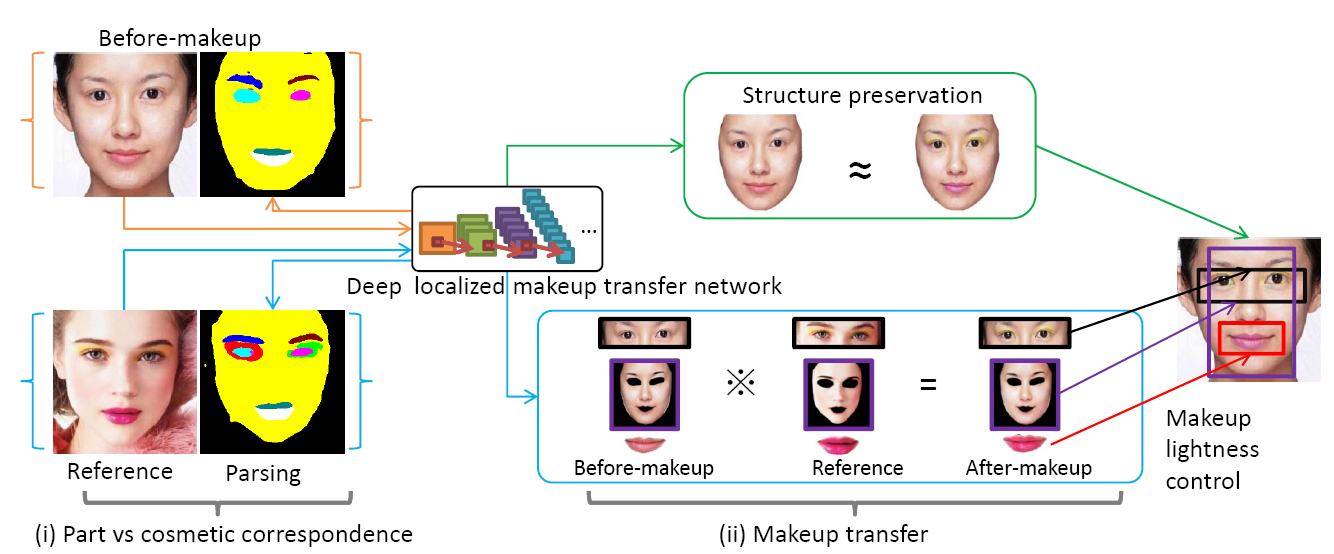
1) With complete functions: we consider three kinds of most commonly used cosmetics, i.e., foundation, eye shadow and lip gloss. Note that our model is quite generic and easily extended to other types of cosmetics.
2) Cometic specific: different cosmetics are transferred in their own ways. For example, maintaining the shape is important for the eye shadow rather than for the foundation.
3) Localized: all cosmetics are applied locally on their corresponding facial parts. For example, lip gloss is put on the lip while eye shadow is worn the eyelid.
4) Naturally looking: the cosmetics need to be seamlessly fused with the before-makeup face. In other words, the after-makeup face should look natural.
5) Controllable makeup lightness: we can change the lightness of each type of cosmetic
Makeup Transfer
In our system, there are four main step: Makeup Recommendation, Face Parsing, Eye shadow Warping, and Makeup Transfer.
1. Makeup Recommendation
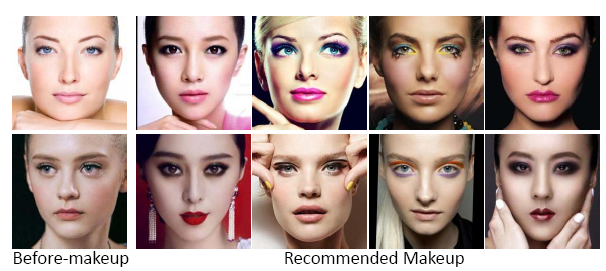
2. Face Parsing
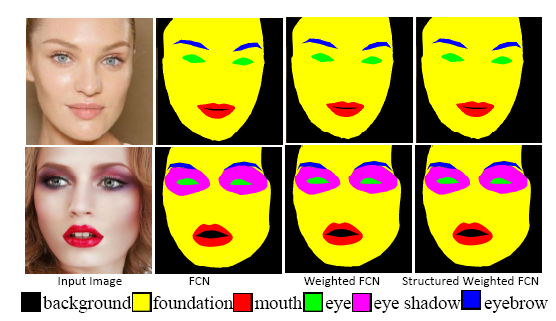
3. Eye Shadow Warping
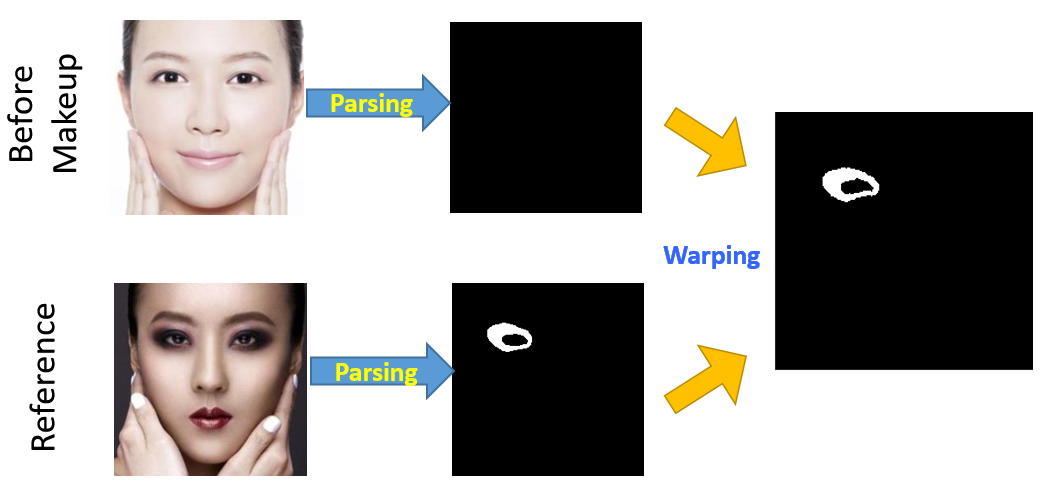
4. Makeup Transfer (eye shadow and lip gloss transfer)
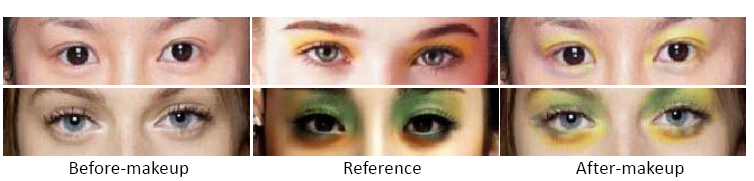
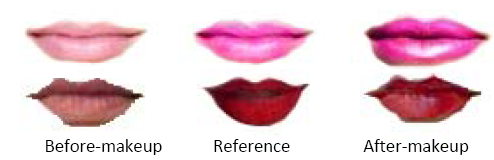
Examples
1. Lightness Control
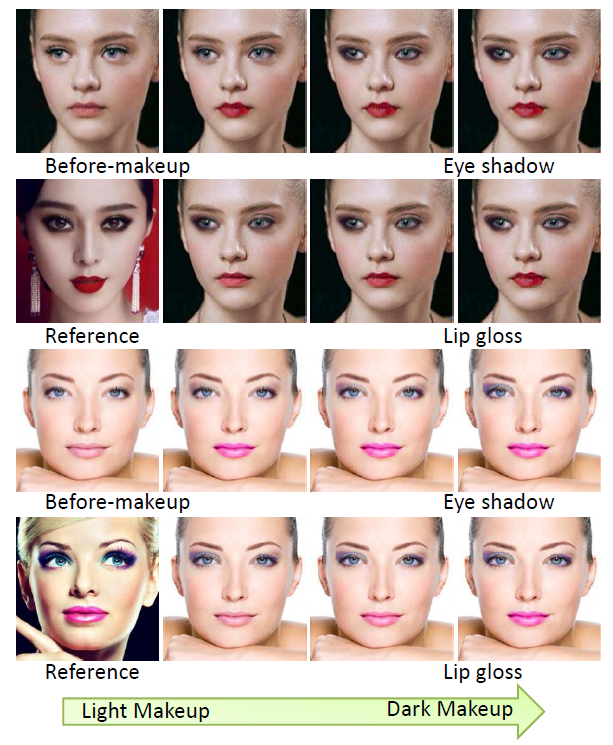
2. Different girls wear the same makeup
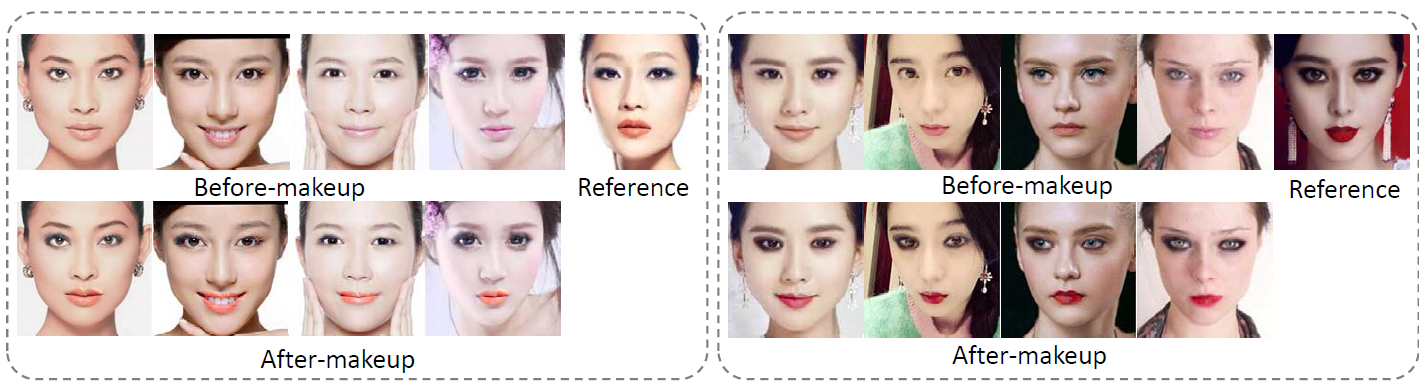
3. The same girl wears different makeups
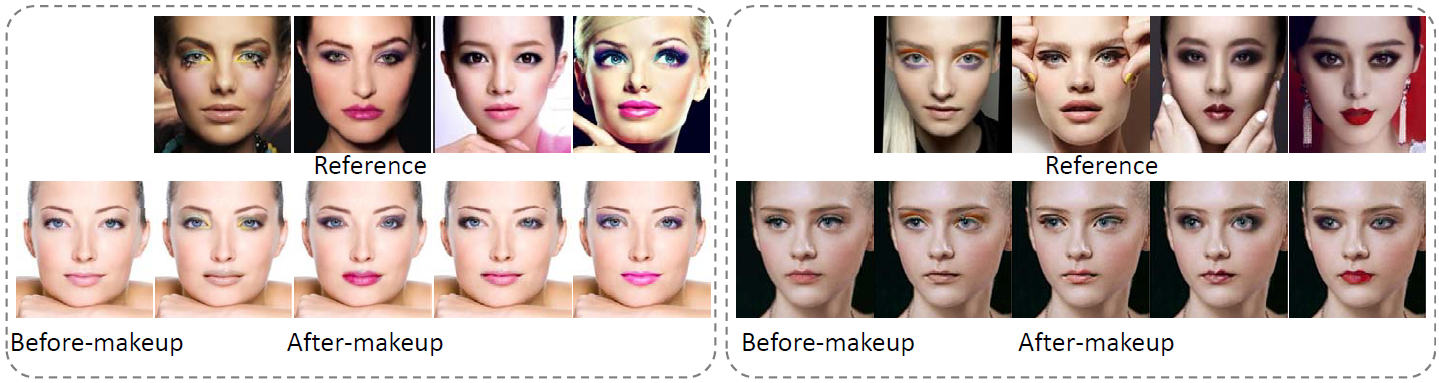
Downloads
1. Si Liu, Xinyu Ou, Ruihe Qian, Wei Wang, Xiaochun Cao. Makeup Like a Superstar: Deep Localized Makeup Transfer Network. The 25th International Joint Conference on Artificial Intelligence (IJCAI-2016). New York City. USA. 9th-15th July, 2016 [arXiv] [bibtex]
2. Xinyu Ou, Si Liu, Xiaochun Cao, Hefei Ling. Beauty eMakeup: A Deep Makeup Transfer System. ACM Multimedia 2016 demo program. Amsterdam, The Netherlands. 15th-19th October, 2016 [Slide]
Reference
[1] Dong Guo and Terence Sim. Digital face makeup by example. Computer Vision and Pattern Recognition, pages 73–79, 2009.
[2] Leon A Gatys, Alexander S Ecker, and Matthias Bethge. A neural algorithm of artistic style. CoRR, abs/1508.06576, 2015.